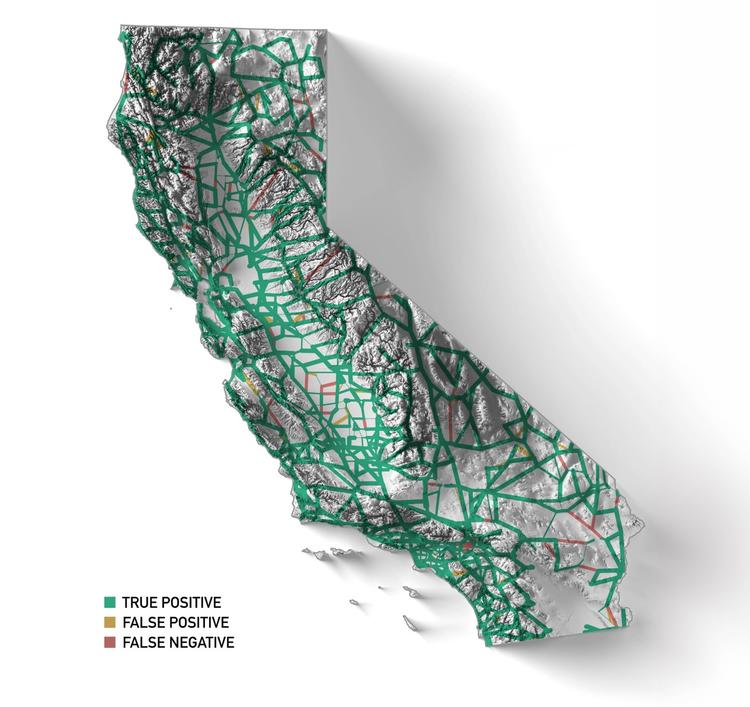
Our latest research, "Modeling of spatially embedded networks via regional spatial graph convolutional networks", is now published and available as open access in CACAIE.
We introduce the Regional Spatial Graph Convolutional Network (RSGCN), a novel geometric-based deep learning model designed to capture complex patterns in spatially embedded networks, such as road networks and power grids. The RSGCN outperforms state-of-the-art models by integrating regional spatial information, achieving up to 38% better accuracy in network reconstruction tasks.
Key Highlights:
- Innovative Approach: Combines regional spatial and vectorized features for more accurate network representation.
- Scalable Solution: Applicable to large-scale networks using a partition-then-ensemble framework.
- Superior Performance: Outperforms models like GraphSAGE and SGCN by up to 38% in network reconstruction tasks.
Applications:
Our model has been rigorously tested on diverse datasets, including synthetic networks, the California Highway Network, and the New Jersey Power Network, demonstrating its versatility and robustness.
This advancement emphasizes the importance of spatial information in modeling complex networks, paving the way for more precise infrastructure analysis.
Discover more about our work and access the open-access paper here.
Plus, our code and data are open-source! Explore them on Zenodo.